Abstract
Aims
Deciphering the genetic relationships between major depressive disorder (MDD) and osteoarthritis (OA) may facilitate an understanding of their biological mechanisms, as well as inform more effective treatment regimens. We aim to investigate the mechanisms underlying relationships between MDD and OA in the context of common genetic variations.
Methods
Linkage disequilibrium score regression was used to test the genetic correlation between MDD and OA. Polygenic analysis was performed to estimate shared genetic variations between the two diseases. Two-sample bidirectional Mendelian randomization analysis was used to investigate causal relationships between MDD and OA. Genomic loci shared between MDD and OA were identified using cross-trait meta-analysis. Fine-mapping of transcriptome-wide associations was used to prioritize putatively causal genes for the two diseases.
Results
MDD has a significant genetic correlation with OA (rg = 0.29) and the two diseases share a considerable proportion of causal variants. Mendelian randomization analysis indicates that genetic liability to MDD has a causal effect on OA (bxy = 0.24) and genetic liability to OA conferred a causal effect on MDD (bxy = 0.20). Cross-trait meta-analyses identified 29 shared genomic loci between MDD and OA. Together with fine-mapping of transcriptome-wide association signals, our results suggest that Estrogen Receptor 1 (ESR1), SRY-Box Transcription Factor 5 (SOX5), and Glutathione Peroxidase 1 (GPX1) may have therapeutic implications for both MDD and OA.
Conclusion
The study reveals substantial shared genetic liability between MDD and OA, which may confer risk for one another. Our findings provide a novel insight into phenotypic relationships between MDD and OA.
Cite this article: Bone Joint Res 2022;11(1):12–22.
Article focus
-
We aim to investigate mechanisms underlying relationships between major depressive disorder (MDD) and osteoarthritis (OA) in the context of common genetic variations.
Key messages
-
Our results indicate that the two diseases have a close genetic correlation and share considerable causal variants.
-
Our Mendelian randomization analysis indicated that genetic liability to MDD and OA confers risk for one another.
-
Cross-trait meta-analyses identified 29 shared genomic loci between MDD and OA.
-
Together with fine-mapping of transcriptome-wide association signals, our results suggest Estrogen Receptor 1 (ESR1), SRY-Box Transcription Factor 5 (SOX5), and Glutathione Peroxidase 1 (GPX1) as promising risk genes for MDD and OA, which may inform treatment regimens for patients who have both diseases.
Strengths and limitations
-
We employed multiple analytic frameworks to systematically elucidate the genetic relationships between MDD and OA.
-
Transcriptome-wide association study associations may potentially contain noises, since the gene expression levels were imputed from weighted linear combinations of single-nucleotide polymorphisms.
Introduction
Major depressive disorder (MDD) is characterized by persistent low mood and is the most prevalent mental disorder accompanied by considerable morbidity, mortality, and high risk of suicide.1 It confers a heavy burden on society, not only due to its high prevalence, but also because of the high comorbidity with other medical outcomes,2 which further worsens the outcome of other health problems and increases mortality. Long-term depression generally adds to the risk for somatic illness, while chronic somatic diseases are frequently accompanied by depression.3
Osteoarthritis (OA) is the most common musculoskeletal disease worldwide, and a leading cause of pain and disability in older adults.4 OA is characterized by progressive cartilage loss, osteophyte formation, and subchondral sclerosis, leading to a large amount of pain and disability in the elderly worldwide.5 A major genetic component to OA risk has been demonstrated by epidemiological studies.6 OA pain and associated disability may increase the risk for MDD through both biological and psychological mechanisms.7 Chronic pain leads to decreased brain volume, specifically for mood regulation,8 and the disability resulting from knee OA may result in psychological changes relevant to depression. According to a report from 2015, approximately 21% of adults with OA coexist with depression.9 OA patients coexisting with depression report higher healthcare costs, and use pain medication more frequently than those without depression.9,10
Although previous studies have detected associations between MDD and OA, key questions remain: 1) to what extent do the two conditions share genetic influences?; 2) are the associations driven by aetiologically causal effects?; and 3) what potential biomarkers or mechanisms may underline these associations?
A genetic correlation coefficient is a prevailing measure to qualify the genetic relationship between two traits. The sign of the correlation coefficient indicates directions of the shared genetic effects. However, genetic correlation analyses may be underpowered in dealing with mixtures of effect directions across shared genetic variants.11 Polygenic overlap was recently proposed to measure the fraction of genetic variants causally associated with both traits over the total number of causal variants across a pair of traits involved.11 Frei et al11 introduced a novel statistical framework (MiXeR) to quantify polygenic overlap irrespective of genetic correlation between traits. In the MiXeR pipeline, the total number of shared and trait-specific causal variants across a pair of traits is quantified.
Mendelian randomization (MR) is an analytic framework that uses genetic variants as instrumental variables to test for causative association between an exposure and an outcome.12 MR is efficient and cost-effective for large datasets curated from genome-wide association studies (GWASs). Recently, GSMR has been developed by leveraging power from multiple genetic variants accounting for linkage disequilibrium (LD) between the variants.13
In this study, we estimated genetic correlation and polygenic overlap between MDD and OA. Pleiotropic genomic loci shared between MDD and OA were identified using cross-trait meta-analyses. We further performed a multi-single-nucleotide polymorphism (SNP) MR analysis on summary results presented in GWAS datasets to test the causal associations between MDD and OA. In addition, we explored potential biological mechanisms underlying the phenotypic relationships between the two diseases.
Methods
GWAS summary datasets and quality control
This study relied on deidentified summary-level data that have been made publically available, and part of the MDD dataset was obtained by approval from 23andMe.14 Ethical approval had been obtained in all original studies. The MDD dataset includes 135,458 cases and 344,901 healthy controls,15 and the OA dataset includes 77,052 cases and 378,169 healthy controls.16 For each dataset, inclusion criteria include bi-allelic SNPs and imputation information above 0.9. Each SNP was compared between two traits and ambiguous SNPs were excluded. If a SNP was mapped in opposite strands in the two datasets, alleles of the SNP in the second dataset were flipped. Effect direction of a SNP was reversed for the second dataset if alleles of the SNP were reversed in the two datasets.
Genetic correlation and polygenic overlap analysis
GWAS summary results were used to analyze the genetic correlation of MDD with OA using linkage disequilibrium (LD) score regression.17,18 Polygenic overlap was analyzed by MiXeR v1.2 (Norway) using default parameters.11 The test statistics of MiXeR take into account effects of LD structure, minor allele frequency (MAF), sample size, cryptic relationships, and sample overlap. The total number of causal variants is reported as 22.6% of the total estimate, which accounts for 90% of SNP heritability for each trait.
MR analysis
Bidirectional causal associations between MDD and OA were inferred using generalized summary data-based Mendelian randomization (GSMR).13 Instrumental variants were selected based on default p ≤ 5 × 10-8. Pleiotropy is a potential source of bias that can lead to an inflated estimation in a MR analysis.19 Therefore, pleiotropy evaluation for a large number of instrumental variants is critical. In GSMR, HEIDI-outlier offers a statistical approach to detect and eliminate genetic instruments with apparent pleiotropic effects on both risk factors and disease.13,20
Cross-trait meta-analysis
We performed a cross-trait meta-analysis of the MDD with OA using the subset-based fixed-effects method ASSET v2.4.0, which permits the characterization of each SNP concerning its pattern of effects on multiple phenotypes.21 The analysis results return a p-value and show the best subset containing the studies contributing to the overall association signal for each variant. The meta-analysis pools the effect of a given SNP across two studies, weighting the effects by the size of the study. After subset-based meta-analysis, SNPs for which two-tailed p-values were lower than 5 × 10-8 were considered statistically significant. Functional mapping and animation (FUMA) was used to map SNPs to genes and identify LD-independent genomic regions.22
To ensure that sample overlap did not contribute to inflated estimates of genetic overlap between MDD and the three traits, λmeta statistics were calculated.23 The λmeta is a statistic that uses effect size concordance to detect sample overlap or heterogeneity. Under the null hypothesis, λmeta = 1 when the pair of cohorts are completely independent. When there are overlapping samples, λmeta < 1.
Fine-mapping of TWAS associations
To prioritize putatively causal genes, we used fine-mapping of causal gene sets (FOCUS v 0.6.10 (USA))24 to the meta-analysis result of MDD and OA in the brain. FOCUS models predicted expression correlations and assign a posterior inclusion probability (PIP) for genes at each transcriptome-wide association study (TWAS) region and relevant tissue types. TWAS has been employed to identify risk genes for OA.25 A multi-tissue expression quantitative trait loci (eQTL) reference weight database from the software was used as eQTL weights, and LD information from linkage disequilibrium score regression (LDSC) was used as reference.
Knowledge-based analysis
We obtained GWAS results (including meta-analysis) of depression (MDD and depressive symptoms) and OA from the GWAS Catalogue database.26,27 Protein-protein interaction analysis was conducted using STRING v11 (Academic Consortium, ELIXIR, UK).28 Specific expression analysis (SEA v1.1; Dougherty Lab, USA) was used to test whether the identified risk genes are over-represented by enriched expression in adult brain regions and development.29,30 For each tissue, transcripts from the processed GTEx transcripts that are specifically expressed or enriched have been identified by using the SEA pSI R package function to calculate the specificity index probability (pSI).29 The significance levels of shared genes between MDD and OA enriched in each tissue were identified by Fisher’s exact test with Benjamini-Hochberg correction. We then explored whether the genes shared by MDD and OA have been implicated in previous genome-wide association studies. A detailed description of the methods is provided in the Methods section of the Supplementary File.
Statistical analysis
Statistical analyses were conducted using R 4.0.5 (R Foundation for Statistical Computing, Austria) or Python 3.8 environment. LD score regression was used to measure genetic correlation between MDD and OA. Bivariate causal mixture model was used to quantify polygenic overlap between MDD and OA. A p-value < 0.05 indicated that the difference was statistically significant.
Results
Genetic correlation and polygenic overlap analysis
Genetic correlation analyses indicated that MDD has a significant genetic correlation with OA (rg = 0.29, standard error (SE) = 0.03, p = 4.11×10-30). Polygenic analysis indicated that 15,800 variants causally influence MDD and 8.9 K influence OA. Among these variants, 5.9 K are shared between the two diseases (Figure 1a).
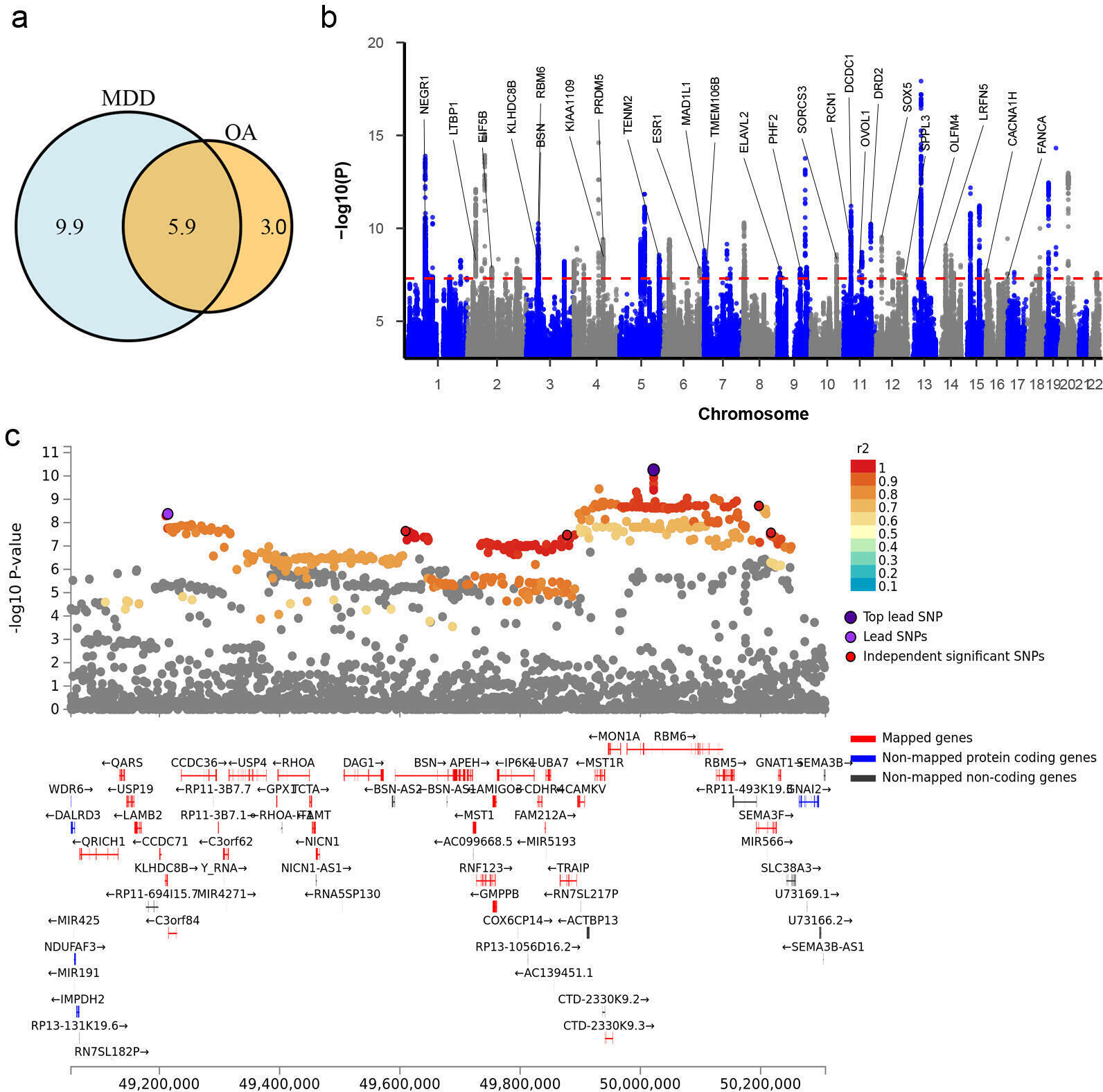
Fig. 1
Shared genetic liability between major depressive disorder (MDD) and osteoarthritis (OA). a) Venn diagrams of unique and shared polygenic components at the causal level, showing the polygenic overlap between MDD and OA. The numbers indicate the estimated quantity of causal variants (in thousands) per component, explaining 90% of single nucleotide polymorphism (SNP) heritability in each phenotype. The size of the circles reflects the degree of polygenicity. b) Manhattan plot of meta-analysis of MDD with OA. The x-axis is the chromosomal position of SNPs and the y-axis is the significance of the SNPs (-log10P). Protein-coding genes containing or adjacent to independent significant SNPs shared by two traits were annotated. c) A genomic locus shared by MDD and OA and implicated by fine-mapping of transcriptome-wide association signals.
MR analysis
MR analysis indicated that genetic liability of MDD conferred a causal effect on OA (bxy = 0.24, s.e. = 0.04, p = 2.36×10-8, Figure 2a) and genetic liability of OA conferred a causal effect on MDD (bxy = 0.20, s.e. = 0.05, p = 2.74×10-5, Figure 2b).
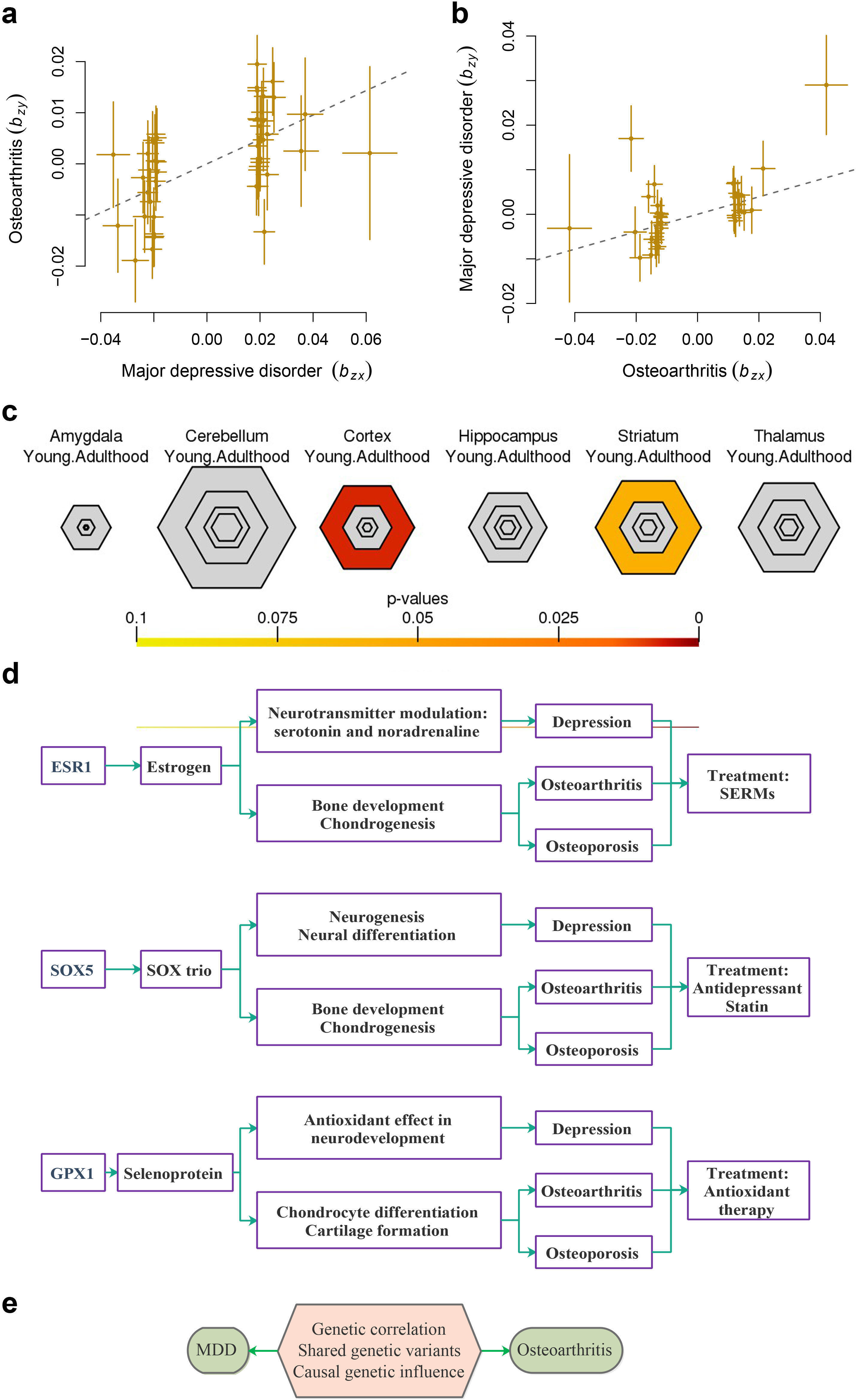
Fig. 2
The associations between major depressive disorder and osteoarthritis and specific expression analyses (SEA). a) and b) The causal associations between major depressive disorder (MDD) and osteoarthritis (OA). The lines denote effect sizes (bxy). c) SEA of adult brain regions for the 25 shared genes. The significance of overlap of provided gene lists with transcripts enriched in specific cell types or tissue types is indicated by the intensity of colour. d) Schematic relationship between Estrogen Receptor 1 (ESR1) and SRY-Box Transcription Factor 5 (SOX5) and depression, OA, and osteoporosis. e) Genetic variation mediating associations between MDD and OA. SERMs, selective oestrogen receptor modulators.
Cross-trait meta-analysis
The cross-trait meta-analysis of MDD and OA revealed 71 loci, 176 independent significant SNPs (IndSigSNPs), and 82 lead SNPs, including 51 pleiotropic IndSigSNPs located in 29 loci (associated with both traits) (Figure 1b, Table I, Supplementary Table i). A total of 75 pleiotropic protein-coding genes were identified, including 25 protein-coding genes which were implicated by the pleiotropic IndSigSNPs and 50 protein-coding genes located within the clumping range of independent significant SNPs (Supplementary Table ii). λmeta values were 1.17 for datasets between MDD and OA, indicating no significant overlap between MDD and the OA GWAS samples. Quantile-quantile (QQ) plots displaying the observed meta-analysis statistics, versus the expected statistics under the null model of no associations in the -log10(p) scale, are shown in Supplementary Figure a.
Table I.
Genomic loci shared between major depressive disorder and osteoarthritis.
Lead SNP | Chr:BP | p-value | Start:End | Genes |
---|---|---|---|---|
rs10789340 | 1:72,940,273 | 1.29 × 10-14 | 72511514:74,077,588 | NEGR1; RPL31P12; RP4-660H19.1; RP11-262K1.1 |
rs2061027 | 2:33,434,336 | 8.05 × 10-13 | 33370457:33,464,969 | LTBP1 |
rs6720885 | 2:99,971,289 | 1.30 × 10-8 | 99573471:100,109,001 | EIF5B |
rs12471530 | 2:215,433,178 | 1.75 × 10-8 | 215407397:215,481,251 | AC107218.3 |
rs199956414 | 3:50,022,089 | 5.51 × 10-11 | 49109919:50,250,837 | KLHDC8B; BSN; RBM6; C3orf84 |
rs13143036 | 4:121,623,038 | 3.99 × 10-10 | 121546342:121,655,414 | PRDM5 |
rs45510091 | 4:123,186,393 | 3.26 × 10-9 | 123122856:123,558,330 | KIAA1109 |
rs1363104 | 5:103,917,797 | 1.46 × 10-12 | 103671867:104,082,179 | RP11-6N13.1 |
rs1549212 | 5:166,996,722 | 4.77 × 10-9 | 166985224:167,055,936 | TENM2 |
rs9479138 | 6:152,215,199 | 1.42 × 10-8 | 152201201:152,264,529 | ESR1 |
rs3823624 | 7:2,110,346 | 1.55 × 10-9 | 1873756:2,110,850 | MAD1L1 |
rs10950398 | 7:12,264,871 | 6.82 × 10-9 | 12233848:12,285,140 | TMEM106B |
rs13246482 | 7:109,794,839 | 1.51 × 10-8 | 109716293:109,794,839 | (No genes mapped) |
rs3793577 | 9:23,737,627 | 4.73 × 10-8 | 23736400:23,737,627 | ELAVL2 |
rs7044244 | 9:96,397,689 | 1.52 × 10-8 | 96349538:96,484,560 | PHF2 |
rs10818400 | 9:122,664,468 | 1.23 × 10-8 | 122655283:122,676,328 | RP11-360A18.2 |
rs61867293 | 10:106,563,924 | 2.59 × 10-9 | 106418969:106,768,514 | SORCS3 |
rs10835766 | 11:31,374,329 | 6.35 × 10-12 | 30750092:31,858,991 | DCDC1; RCN1 |
rs644740 | 11:65,561,468 | 2.10 × 10-8 | 65501060:65,566,719 | OVOL1 |
rs1149620 | 11:76,506,572 | 1.92 × 10-9 | 76464812:76,511,271 | RP11-672A2.1; RP11-21L23.4 |
rs11608185 | 11:113,294,976 | 5.82 × 10-11 | 113236199:113,451,765 | DRD2 |
rs7305875 | 12:23,971,243 | 3.00 × 10-10 | 23929026:24,077,866 | SOX5 |
rs2193743 | 12:108,885,446 | 5.30 × 10-9 | 108878314:108,888,467 | RP11-13G14.4 |
rs73224311 | 12:121,344,656 | 3.68 × 10-8 | 121068253:121,423,742 | SPPL3; CLIC1P1 |
rs12552 | 13:53,625,781 | 1.20 × 10-18 | 53605160:54,056,553 | OLFM4; LINC01065; RN7SL618P; AL450423.1 |
rs1950829 | 14:42,097,937 | 8.10 × 10-10 | 41969803:42,183,025 | LRFN5 |
rs8037355 | 15:37,643,831 | 6.57 × 10-13 | 37581276:37,840,264 | RP11-597G23.1; RP11-720L8.1 |
rs191117454 | 16:1,249,053 | 2.06 × 10-8 | 1246747:1,255,390 | CACNA1H |
rs1126464 | 16:89,704,365 | 3.62 × 10-10 | 89669631:89,857,431 | FANCA |
-
BP, base position; Chr, chromosome.
Fine-mapping of TWAS associations
We used fine-mapping of TWAS associations to prioritize putatively causal genes from the meta-analysis of MDD and OA. A total of 81 gene-tissue pairs were identified as in the 90% credible set in the brain tissue, involving 80 genes (Supplementary Table iv). Nine genes in the credible set with high PIP (> 0.90) are shown in Table II.
Table II.
Fine-mapping transcriptome-wide association signals in brain.
Gene | Tissue | TWAS_Z | PIP | Region |
---|---|---|---|---|
RPL31P12 | brain_cerebellum | -7.61 | 1 | 1:71684831-1:74,326,484 |
UQCC | brain_dorsolateral_prefrontal_cortex | 6.75 | 1 | 20:32813689-20:34,960,446 |
AXIN1 | brain_cerebellar_hemisphere | -5.74 | 1 | 16:1207833-16:2,763,816 |
ZNF184 | brain_hypothalamus | -5.13 | 1 | 6:25684587-6:26,789,628 |
DNAJC24 | brain_dorsolateral_prefrontal_cortex | 5.33 | 0.99 | 11:30141357-11:32,276,662 |
FAM86B3P | brain_cerebellar_hemisphere | -5.28 | 0.99 | 8:7153384-8:9,154,694 |
GPX1 | brain_frontal_cortex_ba9 | 5.99 | 0.95 | 3:47727379-3:49,316,164 |
PRSS16 | brain_cerebellum | -5.33 | 0.92 | 6:28018353-6:28,917,091 |
HIST1H4H | brain_dorsolateral_prefrontal_cortex | 3.98 | 0.91 | 6:25684587-6:26,789,628 |
-
PIP, posterior inclusion probability; TWAS, transcriptome-wide association study.
Knowledge-based analysis
A total of 17 genes out of the 25 pleiotropic protein-coding genes have been identified in previous GWASs on depression or OA (Supplementary Table iii). At pSI threshold of 0.05, the 25 pleiotropic protein-coding genes were enriched in cortex (false discovery rate (FDR) = 0.007) and marginally enriched in striatum (FDR = 0.058) (Figure 2c). PPI analysis showed that most of the 75 genes were interconnected, constituting two large networks, with ESR1 being involved in one network and SOX5 being involved in another network (Figure 3).
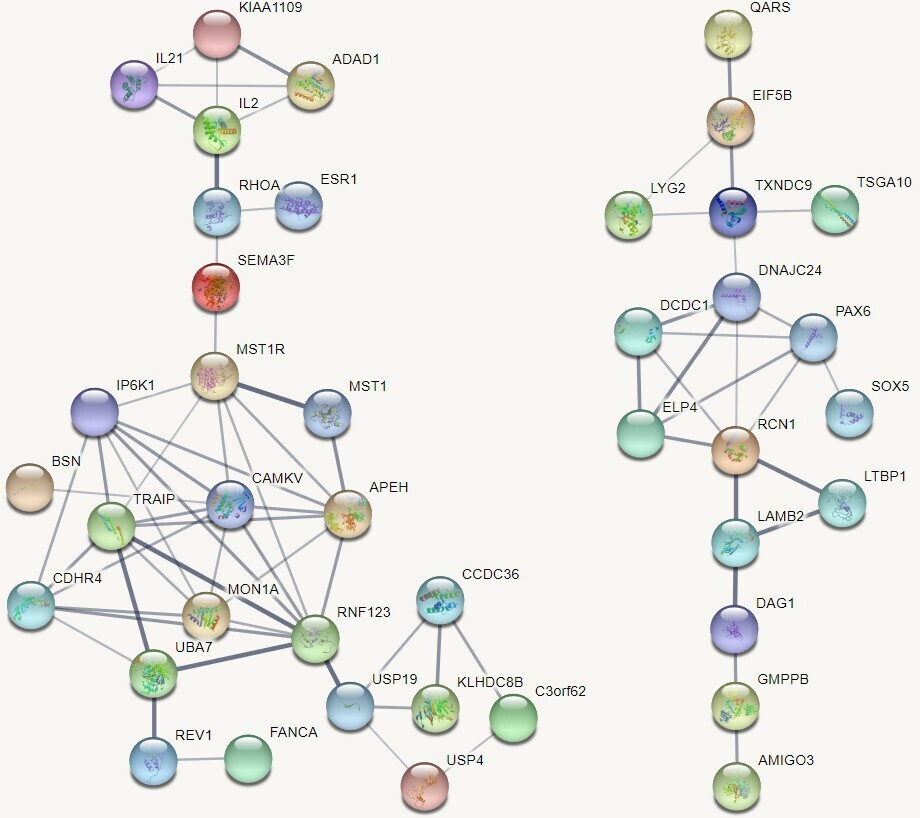
Fig. 3
Protein-protein interactions among the shared genes. These were pleiotropic protein-coding genes related to both major depressive disorder and osteoarthritis.
Discussion
The comorbidity between depression and a myriad of health outcomes typically forms a vicious cycle, known to significantly impact the course and management of depression and its associated conditions. OA, the commonest form of arthritides, is one of the leading causes of functional disability and reduced quality of life worldwide, particularly in the elderly. A better understanding of the pathophysiology of OA is necessary to improve effective preventive strategies.
In this study, we detected a significant genetic correlation between MDD and OA (r = 0.29), higher than those between MDD and autism spectrum disorder (r = 0.16) and obsessive-compulsive disorder (r = 0.23).31 More than half (66%) of causal variants influencing OA risk may also affect MDD. Our results indicate a higher polygenicity of MDD than OA. More importantly, we identified the bidirectional causal effects between MDD and OA, indicating that the liability of depression and OA may aetiologically confer risk on one another. These complementary lines of evidence reveal novel mechanisms underlying phenotypic relationships between MDD and OA (Figure 2e).
Close links between MDD and OA have been well documented.32 However, biological pathways mediating relationships between MDD and OA remain largely elusive. MDD and OA belong to two distinctive medical categories, therefore common genes shared between MDD and OA have rarely been systematically investigated. As yet, only a limited number of genome-wide candidate genes have been reported by GWASs and transcriptome studies on OA.16,33-35 Our cross-trait analysis revealed 29 loci and 75 protein-coding genes shared between MDD and OA. The pleiotropic genes may at least partially mediate the cross-talk between MDD and OA in the context of disease pathogenesis.
Among the 25 protein-coding genes directly implicated by independent significant SNPs, a total of 15 genes were previously identified as genome-wide risk genes for depression, including DCDC1, DRD2, ELAVL2, KIAA1109, KLHDC8B, LRFN5, MAD1L1, NEGR1, OLFM4, PHF2, SORCS3, SOX5, SPPL3, TENM2, and TMEM106B. However, the majority of the pleiotropic genes identified are novel risk genes at the genome-wide level for OA, except for two genes, LTBP1 and RBM6. Our study sheds new light on the genetic susceptibility of OA and MDD. Some of these genes may have implications for treatment regimens for patients comorbid with the two diseases.
Accumulating evidence indicates the involvement of oestrogen in depression.36 Depression is associated with altered levels of neurotransmitters and abnormal functioning of the hypothalamic-pituitary-adrenal axis. Oestrogen can modulate neurotransmitter turnover to enhance the levels of serotonin and noradrenaline, and is involved in the regulation of serotonin receptor number and function.37 Fluctuating oestrogen levels during the female reproductive life are associated with depressed mood.38 Oestrogen exerts its biological effects chiefly through intracellular activation of oestrogen receptor α (ESR1) and oestrogen receptor β (ESR2).
ESR1 belongs to the nuclear receptor superfamily of ligand-regulated transcription factors. ESR1 has been reported to be associated with MDD39 and perinatal depression.40,41ESR1 was implicated in anxiety-like behaviour and was identified to be a genome-wide risk gene for anxiety.42 Associations between ESR1 and MDD could have useful preventive and therapeutic implications, and help to lead to more personalized therapies based on one’s genetic profile. Neonatal treatment with antidepressant clomipramine in rats induces changes in oestrogen receptors in different brain areas involved with the regulation of depressive-like behaviours.43 A beneficial effect of oestrogen-containing hormone treatment (HT) has been reported in depressed peri-menopausal and postmenopausal women.44,45
Oestrogen and its receptors are essential for sexual development and reproductive function, but also play a role in other tissues such as bone. ESR1 is expressed in chondrocytes, stromal cells, and osteoblasts,46 indicating its potential role in OA. Genetic variants within ESR1 have been reported to be associated with OA,47,48 and were suggested to be one of the promising risk genes for OA.6,49ESR1 has been repeatedly identified as a genome-wide risk gene for bone mineral density,50-53 demonstrating its vital role in osteoporosis and fractures.6
The presence of oestrogen receptors in joint tissues and the increased prevalence of OA after menopause suggests the potential value of oestrogen treatment in OA patients. Oestrogen-related agents may exert an effect on subchondral bone and the surrounding tissues, including the articular cartilage, synovium, and muscle. Recent studies have suggested that oestrogen or selective oestrogen receptor modulators (SERMs) may exert a beneficial effect in OA with relative safety and tolerability profiles.54,55 SERMs like raloxifene and bazedoxifene have been approved for the treatment of osteoporosis.55 SERMs may be particularly beneficial for postmenopausal patients with early-stage OA or osteoporotic OA.54,55 This cross-trait meta-analysis suggests ESR1 as a novel genome-wide risk gene for both MDD and OA, corroborating its role in the aetiology of both OA and MDD (Figure 2d). We postulate that oestrogen or SERMs may be favourable for patients comorbid with depression and OA.
The SOX5 gene encodes one of the SOX family of transcription factors involved in the regulation of cell fate and differentiation in neurogenesis and other discrete developmental processes.56,57SOX5 haploinsufficiency leads to the neurodevelopmental disorder Lamb-Shaffer syndrome.58SOX5 was a genome-wide risk gene for MDD,15,59,60 and was associated with response to antidepressant61 and antipsychotics.62
SOX5 is one member of the SOX trio (SOX5, SOX6, and SOX9) that is crucial for the development of primordial cartilage and chondrogenesis.63,64 In addition, SOX5 was a genome-wide gene for heel bone mineral density and may be involved in osteoporosis.52,65,66 Our study identified SOX5 as a novel genome-wide risk gene for OA, providing additional evidence for its involvement in OA. However, further studies are warranted to elucidate the mechanisms of SOX5 in the pathogenesis of MDD.
Empirical evidence has shown that SOX5 was associated with response to statin.67 Statins are used widely in primary and secondary prevention of cardiovascular disease due to their cholesterol-lowering properties. Statin has been reported to have beneficial effects on OA,68 especially knee OA.69,70 Statin use is associated with decreased risks of osteoporosis, hip fracture, and vertebral fracture in stroke patients.71 A meta-analysis indicated that statin treatment may be associated with a decreased risk of overall fractures and hip fractures, and increased bone mineral density and osteocalcin.72
Statins also have anti-inflammatory effects independent of their lipid-lowering mechanisms. Low-grade inflammation is repeatedly observed in depression patients, and anti-inflammatory drugs have shown antidepressant actions. Studies have suggested that statin use is associated with a reduced risk of MDD,73 which may be explained by the anti-inflammatory properties of statin.74 It was reported that concomitant treatment with SSRIs and statins leads to better response compared with SSRIs alone.75,76 It is suggested that statins used in combination with psychotropic medications may be effective for various psychiatric conditions, including depression, schizophrenia, and dementia.77 Antidepressants have also been used in OA patients. A meta-analysis indicated that duloxetine has moderate benefits on pain, function, and quality of life in knee OA patients.78 Therefore, it is tempting to speculate that statins may be more effective to be used as an add-on treatment to an antidepressant for patients with comorbid depression and OA/osteoporosis (Figure 2d).
To identify potentially causal genes involved in MDD and OA, we used the fine-mapping of TWAS hits to estimate the causality in the brain tissue. A total of 80 genes were in the 90%-credible set, including nine genes with high PIP. Three of the nine genes are shared by MDD and OA in our meta-analysis, including RPL31P12, DNAJC24, and GPX1. The RPL31P12 gene, located in 1p31.1, is a pseudogene with unknown functions. The DNAJC24 gene maps to 11p13, and its function is not fully understood. It has been reported that silencing of DNAJC24 gene transcription is associated with immunotoxin resistance.79
GPX1 in the 3p21.31 region was included in the credible gene set with a PIP of 0.95 in the left ventricle of the heart (Figure 1c). GPX1 encodes a selenium-containing protein that catalyzes the reduction of organic hydroperoxides and hydrogen peroxide by glutathione, and protects cells against oxidative damage. Previous studies indicated that antioxidant disturbances play a vital role in the pathogenesis of neurodegenerative disorders, including depression.80 Expression levels of GPX1 were lower in oligodendrocytes from MDD donors as compared to control donors, which may account for shortened telomere length in these patients.81 Decreased levels of GPX1 were associated with depression severity in the patients.82 Animal studies showed that the expression of GPX1 in the brain changed following chronic mild stress or venlafaxine exposure.83 A previous GWAS has implicated GPX1 in depression.59
The phenotypes of OA consist of disturbance of cartilage extracellular matrix (ECM) metabolism and the imbalance of cartilage homeostasis resulting from pro-inflammatory factors and oxidative stress. GPX1 is a major antioxidant enzyme in osteoclasts and is highly expressed in chondrocytes.84 GPX1 plays a role in chondrocyte differentiation and cartilage formation.85 It was reported that GPX1 messenger RNA (mRNA) expression was lower in damaged cartilage than in smooth cartilage from the OA patients.86 It was reported that bone marrow-derived stem cell-treated ataxic mice showed higher levels of catalase and GPX1.87 Oestrogen is a vital regulator in maintaining bone health, and GPX1 is upregulated by oestrogen.88 Therefore, our study links the cross-talk between GPX1 and oestrogen to the pathogenesis of both depression and OA (Figure 2d).
Shared genetic liability between MDD and OA offers the potential to evaluate osteoarthritic risk in MDD patients and to evaluate the risk to develop depression in OA patients, using strategies like polygenic risk scores. Identification of shared genetic basis between MDD and OA may guide drug discovery, and inform early prediction and personalized treatment for the comorbidities. Since medical comorbidities contribute to poor treatment effects, we argue that it may be beneficial to incorporate screening of depression into the treatment regimen for OA, since improved management may be achieved with add-on psychological or psychiatric interventions for subgroups with higher depression.
The presented study has several strengths. First, we typically prioritized the largest available datasets as a study backbone. Furthermore, to avoid potential population heterogeneity across the studies, we limited our analysis to individuals of European ancestry. Lastly, we employed multiple analytic frameworks to systematically elucidate the genetic relationships between MDD and OA.
However, several limitations should be noted. As our analysis was limited to a genetic component of each trait, the presented results should be interpreted cautiously, with the understanding that human traits result from a complex web of interactions among a plethora of psycho-social-environmental factors. We could not exclude the possibility that some patients with OA may have comorbid depression, which may bias the result. TWAS associations may potentially contain noises, since the gene expression levels were imputed from weighted linear combinations of SNPs. Due to the lack of an eQTL reference dataset in bone and joints, the causality of genes could not be evaluated using TWAS signals for OA.
In summary, our findings indicated that MDD and OA share substantial genetic liability, which may also confer risk on one another. Our study reveals novel mechanisms underlying phenotypic relationships between MDD and OA.
References
1. Ferrari AJ , Charlson FJ , Norman RE , et al. Burden of depressive disorders by country, sex, age, and year: findings from the global burden of disease study 2010 . PLoS Med . 2013 ; 10 ( 11 ): e1001547 . Crossref PubMed Google Scholar
2. Gili M , Garcia-Toro M , Vives M , et al. Medical comorbidity in recurrent versus first-episode depressive patients . Acta Psychiatr Scand . 2011 ; 123 ( 3 ): 220 – 227 . Crossref PubMed Google Scholar
3. Veerman JL , Dowrick C , Ayuso-Mateos JL , Dunn G , Barendregt JJ . Population prevalence of depression and mean Beck Depression Inventory score . Br J Psychiatry . 2009 ; 195 ( 6 ): 516 – 519 . Crossref PubMed Google Scholar
4. O’Neill TW , McCabe PS , McBeth J . Update on the epidemiology, risk factors and disease outcomes of osteoarthritis . Best Pract Res Clin Rheumatol . 2018 ; 32 ( 2 ): 312 – 326 . Crossref PubMed Google Scholar
5. Zhuo Q , Yang W , Chen J , Wang Y . Metabolic syndrome meets osteoarthritis . Nat Rev Rheumatol . 2012 ; 8 ( 12 ): 729 – 737 . Crossref PubMed Google Scholar
6. Peach CA , Carr AJ , Loughlin J . Recent advances in the genetic investigation of osteoarthritis . Trends Mol Med . 2005 ; 11 ( 4 ): 186 – 191 . Crossref PubMed Google Scholar
7. Karp JF , Dew MA , Wahed AS , et al. Challenges and solutions for depression prevention research: methodology for a depression prevention trial for older adults with knee arthritis and emotional distress . Am J Geriatr Psychiatry . 2016 ; 24 ( 6 ): 433 – 443 . Crossref PubMed Google Scholar
8. Apkarian AV , Sosa Y , Sonty S , et al. Chronic back pain is associated with decreased prefrontal and thalamic gray matter density . J Neurosci . 2004 ; 24 ( 46 ): 10410 – 10415 . Crossref PubMed Google Scholar
9. Agarwal P , Sambamoorthi U . Healthcare expenditures associated with depression among individuals with osteoarthritis: post-regression linear decomposition approach . J Gen Intern Med . 2015 ; 30 ( 12 ): 1803 – 1811 . Crossref PubMed Google Scholar
10. Sale JEM , Gignac M , Hawker G . The relationship between disease symptoms, life events, coping and treatment, and depression among older adults with osteoarthritis . J Rheumatol . 2008 ; 35 ( 2 ): 335 – 342 . PubMed Google Scholar
11. Frei O , Holland D , Smeland OB , et al. Bivariate causal mixture model quantifies polygenic overlap between complex traits beyond genetic correlation . Nat Commun . 2019 ; 10 ( 1 ): 2417 . Crossref PubMed Google Scholar
12. Lawlor DA , Harbord RM , Sterne JAC , Timpson N , Davey Smith G . Mendelian randomization: using genes as instruments for making causal inferences in epidemiology . Stat Med . 2008 ; 27 ( 8 ): 1133 – 1163 . Crossref PubMed Google Scholar
13. Zhu Z , Zheng Z , Zhang F , et al. Causal associations between risk factors and common diseases inferred from GWAS summary data . Nat Commun . 2018 ; 9 ( 1 ): 224 . Crossref PubMed Google Scholar
14. No authors listed . 23andMe . https://www.23andme.com/en-gb ( date last accessed 2 December 2021 ). Crossref PubMed Google Scholar
15. Wray NR , Ripke S , Mattheisen M , et al. Genome-wide association analyses identify 44 risk variants and refine the genetic architecture of major depression . Nat Genet . 2018 ; 50 ( 5 ): 668 – 681 . Crossref PubMed Google Scholar
16. Tachmazidou I , Hatzikotoulas K , Southam L , et al. Identification of new therapeutic targets for osteoarthritis through genome-wide analyses of UK Biobank data . Nat Genet . 2019 ; 51 ( 2 ): 230 – 236 . Crossref PubMed Google Scholar
17. Bulik-Sullivan BK , Loh PR , Finucane HK , et al. LD Score regression distinguishes confounding from polygenicity in genome-wide association studies . Nat Genet . 2015 ; 47 ( 3 ): 291 – 295 . Crossref PubMed Google Scholar
18. Bulik-Sullivan B , Finucane HK , Anttila V , et al. An atlas of genetic correlations across human diseases and traits . Nat Genet . 2015 ; 47 ( 11 ): 1236 – 1241 . Crossref PubMed Google Scholar
19. Solovieff N , Cotsapas C , Lee PH , Purcell SM , Smoller JW . Pleiotropy in complex traits: challenges and strategies . Nat Rev Genet . 2013 ; 14 ( 7 ): 483 – 495 . Crossref PubMed Google Scholar
20. Ong JS , MacGregor S . Implementing MR-PRESSO and GCTA-GSMR for pleiotropy assessment in Mendelian randomization studies from a practitioner’s perspective . Genet Epidemiol . 2019 ; 43 ( 6 ): 609 – 616 . Google Scholar
21. Bhattacharjee S , Rajaraman P , Jacobs KB , et al. A subset-based approach improves power and interpretation for the combined analysis of genetic association studies of heterogeneous traits . Am J Hum Genet . 2012 ; 90 ( 5 ): 821 – 835 . Crossref PubMed Google Scholar
22. Watanabe K , Taskesen E , van Bochoven A , Posthuma D . Functional mapping and annotation of genetic associations with FUMA . Nat Commun . 2017 ; 8 ( 1 ): 1826 . Crossref PubMed Google Scholar
23. Chen GB , Lee SH , Zhu ZX , Benyamin B , Robinson MR . EigenGWAS: finding loci under selection through genome-wide association studies of eigenvectors in structured populations . Heredity (Edinb) . 2016 ; 117 ( 1 ): 51 – 61 . Crossref PubMed Google Scholar
24. Mancuso N , Freund MK , Johnson R , et al. Probabilistic fine-mapping of transcriptome-wide association studies . Nat Genet . 2019 ; 51 ( 4 ): 675 – 682 . Crossref PubMed Google Scholar
25. Qi X , Yu F , Wen Y , et al. Integration of transcriptome-wide association study and messenger RNA expression profile to identify genes associated with osteoarthritis . Bone Joint Res . 2020 ; 9 ( 3 ): 130 – 138 . Crossref PubMed Google Scholar
26. No authors listed . GWAS Catalog . https://www.ebi.ac.uk/gwas ( date last accessed 17 April 2020 ). Google Scholar
27. Buniello A , MacArthur JAL , Cerezo M , et al. The NHGRI-EBI GWAS Catalog of published genome-wide association studies, targeted arrays and summary statistics 2019 . Nucleic Acids Res . 2019 ; 47 ( D1 ): D1005 – D1012 . Crossref PubMed Google Scholar
28. Szklarczyk D , Gable AL , Lyon D , et al. STRING v11: protein-protein association networks with increased coverage, supporting functional discovery in genome-wide experimental datasets . Nucleic Acids Res . 2019 ; 47 ( D1 ): D607 – D613 . Crossref PubMed Google Scholar
29. Dougherty JD , Schmidt EF , Nakajima M , Heintz N . Analytical approaches to RNA profiling data for the identification of genes enriched in specific cells . Nucleic Acids Res . 2010 ; 38 ( 13 ): 4218 – 4230 . Crossref PubMed Google Scholar
30. Xu X , Wells AB , O’Brien DR , Nehorai A , Dougherty JD . Cell type-specific expression analysis to identify putative cellular mechanisms for neurogenetic disorders . J Neurosci . 2014 ; 34 ( 4 ): 1420 – 1431 . Crossref PubMed Google Scholar
31. Brainstorm Consortium , Anttila V , Bulik-Sullivan B , et al. Analysis of shared heritability in common disorders of the brain . Science . 2018 ; 360 ( 6395 ): eaap8757 . Crossref PubMed Google Scholar
32. Sharma A , Kudesia P , Shi Q , Gandhi R . Anxiety and depression in patients with osteoarthritis: impact and management challenges . Open Access Rheumatol . 2016 ; 8 : 103 – 113 . Crossref PubMed Google Scholar
33. Styrkarsdottir U , Lund SH , Thorleifsson G , et al. Meta-analysis of Icelandic and UK data sets identifies missense variants in SMO, IL11, COL11A1 and 13 more new loci associated with osteoarthritis . Nat Genet . 2018 ; 50 ( 12 ): 1681 – 1687 . Crossref PubMed Google Scholar
34. Zengini E , Hatzikotoulas K , Tachmazidou I , et al. Genome-wide analyses using UK Biobank data provide insights into the genetic architecture of osteoarthritis . Nat Genet . 2018 ; 50 ( 4 ): 549 – 558 . Crossref PubMed Google Scholar
35. Li H , Yang HH , Sun ZG , Tang HB , Min JK . Whole-transcriptome sequencing of knee joint cartilage from osteoarthritis patients . Bone Joint Res . 2019 ; 8 ( 7 ): 290 – 303 . Crossref PubMed Google Scholar
36. Ancelin ML , Scali J , Ritchie K . Hormonal therapy and depression: are we overlooking an important therapeutic alternative? J Psychosom Res . 2007 ; 62 ( 4 ): 473 – 485 . Crossref PubMed Google Scholar
37. Summer BE , Fink G . Estrogen increases the density of 5-hydroxytryptamine(2A) receptors in cerebral cortex and nucleus accumbens in the female rat . J Steroid Biochem Mol Biol . 1995 ; 54 ( 1–2 ): 15 – 20 . Crossref PubMed Google Scholar
38. Soares CN , Zitek B . Reproductive hormone sensitivity and risk for depression across the female life cycle: a continuum of vulnerability? J Psychiatry Neurosci . 2008 ; 33 ( 4 ): 331 – 343 . PubMed Google Scholar
39. Ryan J , Scali J , Carrière I , et al. Estrogen receptor alpha gene variants and major depressive episodes . J Affect Disord . 2012 ; 136 ( 3 ): 1222 – 1226 . Crossref PubMed Google Scholar
40. Tan EC , Lim HW , Chua TE , Tan HS , Lee TM , Chen HY . vestigation of variants in estrogen receptor genes and perinatal depression . Neuropsychiatr Dis Treat . 2018 ; 14 : 919 – 925 . Google Scholar
41. Elwood J , Murray E , Bell A , Sinclair M , Kernohan WG , Stockdale J . A systematic review investigating if genetic or epigenetic markers are associated with postnatal depression . J Affect Disord . 2019 ; 253 : 51 – 62 . Crossref PubMed Google Scholar
42. Levey DF , Gelernter J , Polimanti R , et al. Reproducible genetic risk loci for anxiety: results from ∼200,000 participants in the million veteran program . Am J Psychiatry . 2020 ; 177 ( 3 ): 223 – 232 . Crossref PubMed Google Scholar
43. Limón-Morales O , Arteaga-Silva M , Rojas-Castañeda JC , et al. Neonatal treatment with clomipramine modifies the expression of estrogen receptors in brain areas of male adult rats . Brain Res . 2019 ; 1724 : 146443 . Crossref PubMed Google Scholar
44. Zweifel JE , O’Brien WH . A meta-analysis of the effect of hormone replacement therapy upon depressed mood . Psychoneuroendocrinology . 1997 ; 22 ( 3 ): 189 – 212 . Crossref PubMed Google Scholar
45. Gleason CE , Dowling NM , Wharton W , et al. Effects of hormone therapy on cognition and mood in recently postmenopausal women: findings from the Randomized, Controlled KEEPS-Cognitive and Affective Study . PLoS Med . 2015 ; 12 ( 6 ): e1001833; discussion e1001833 . Crossref PubMed Google Scholar
46. Oshima Y , Matsuda KI , Yoshida A , Watanabe N , Kawata M , Kubo T . Localization of estrogen receptors alpha and beta in the articular surface of the rat femur . Acta Histochem Cytochem . 2007 ; 40 ( 1 ): 27 – 34 . Crossref PubMed Google Scholar
47. Riancho JA , García-Ibarbia C , Gravani A , et al. Common variations in estrogen-related genes are associated with severe large-joint osteoarthritis: a multicenter genetic and functional study . Osteoarthritis and Cartilage . 2010 ; 18 ( 7 ): 927 – 933 . Crossref PubMed Google Scholar
48. Ahrar H , Aghili K , Sobhan MR , Mahdinezhad-Yazdi M , Akbarian-Bafghi MJ , Neamatzadeh H . Association of rs2234693 and rs9340799 polymorphisms of estrogen Receptor-1 gene with radiographic defined knee osteoarthritis: A meta-analysis . J Orthop . 2019 ; 16 ( 3 ): 234 – 240 . Crossref PubMed Google Scholar
49. Ryder JJ , Garrison K , Song F , et al. Genetic associations in peripheral joint osteoarthritis and spinal degenerative disease: a systematic review . Ann Rheum Dis . 2008 ; 67 ( 5 ): 584 – 591 . Crossref PubMed Google Scholar
50. Styrkarsdottir U , Halldorsson BV , Gretarsdottir S , et al. Multiple genetic loci for bone mineral density and fractures . N Engl J Med . 2008 ; 358 ( 22 ): 2355 – 2365 . Crossref PubMed Google Scholar
51. Rivadeneira F , Styrkársdottir U , Estrada K , et al. Twenty bone-mineral-density loci identified by large-scale meta-analysis of genome-wide association studies . Nat Genet . 2009 ; 41 ( 11 ): 1199 – 1206 . Crossref PubMed Google Scholar
52. Morris JA , Kemp JP , Youlten SE , et al. An atlas of genetic influences on osteoporosis in humans and mice . Nat Genet . 2019 ; 51 ( 2 ): 258 – 266 . Crossref PubMed Google Scholar
53. Wang H , Zhang F , Zeng J , et al. Genotype-by-environment interactions inferred from genetic effects on phenotypic variability in the UK Biobank . Sci Adv . 2019 ; 5 ( 8 ): eaaw3538 . Crossref PubMed Google Scholar
54. Lugo L , Villalvilla A , Largo R , Herrero-Beaumont G , Roman-Blas JA . Selective estrogen receptor modulators (SERMs): new alternatives for osteoarthritis? Maturitas . 2014 ; 77 ( 4 ): 380 – 384 . Crossref PubMed Google Scholar
55. Xiao YP , Tian FM , Dai MW , Wang WY , Shao LT , Zhang L . Are estrogen-related drugs new alternatives for the management of osteoarthritis? Arthritis Res Ther . 2016 ; 18 : 151 . Crossref PubMed Google Scholar
56. Lefebvre V . The SoxD transcription factors--Sox5, Sox6, and Sox13--are key cell fate modulators . Int J Biochem Cell Biol . 2010 ; 42 ( 3 ): 429 – 432 . Crossref PubMed Google Scholar
57. Naudet N , Moutal A , Vu HN , et al. Transcriptional regulation of CRMP5 controls neurite outgrowth through Sox5 . Cell Mol Life Sci . 2018 ; 75 ( 1 ): 67 – 79 . Crossref PubMed Google Scholar
58. Zawerton A , Mignot C , Sigafoos A , et al. Widening of the genetic and clinical spectrum of Lamb-Shaffer syndrome, a neurodevelopmental disorder due to SOX5 haploinsufficiency . Genet Med . 2020 ; 22 ( 3 ): 524 – 537 . Crossref PubMed Google Scholar
59. Nagel M , Jansen PR , Stringer S , et al. Meta-analysis of genome-wide association studies for neuroticism in 449,484 individuals identifies novel genetic loci and pathways . Nat Genet . 2018 ; 50 ( 7 ): 920 – 927 . Crossref PubMed Google Scholar
60. Howard DM , Adams MJ , Clarke TK , et al. Genome-wide meta-analysis of depression identifies 102 independent variants and highlights the importance of the prefrontal brain regions . Nat Neurosci . 2019 ; 22 ( 3 ): 343 – 352 . Crossref PubMed Google Scholar
61. Fabbri C , Tansey KE , Perlis RH , et al. New insights into the pharmacogenomics of antidepressant response from the GENDEP and STAR*D studies: rare variant analysis and high-density imputation . Pharmacogenomics J . 2018 ; 18 ( 3 ): 413 – 421 . Crossref PubMed Google Scholar
62. Adkins DE , Aberg K , McClay JL , et al. Genomewide pharmacogenomic study of metabolic side effects to antipsychotic drugs . Mol Psychiatry . 2011 ; 16 ( 3 ): 321 – 332 . Crossref PubMed Google Scholar
63. Ikeda T , Kamekura S , Mabuchi A , et al. The combination of SOX5, SOX6, and SOX9 (the SOX trio) provides signals sufficient for induction of permanent cartilage . Arthritis Rheum . 2004 ; 50 ( 11 ): 3561 – 3573 . Crossref PubMed Google Scholar
64. Akiyama H . Control of chondrogenesis by the transcription factor Sox9 . Mod Rheumatol . 2008 ; 18 ( 3 ): 213 – 219 . Crossref PubMed Google Scholar
65. Wen Y , Guo X , Hao J , et al. Integrative analysis of genome-wide association studies and gene expression profiles identified candidate genes for osteoporosis in Kashin-Beck disease patients . Osteoporos Int . 2016 ; 27 ( 3 ): 1041 – 1046 . Crossref PubMed Google Scholar
66. Xu L , Zheng L , Wang Z , et al. TNF-α-Induced SOX5 Upregulation Is Involved in the Osteogenic Differentiation of Human Bone Marrow Mesenchymal Stem Cells Through KLF4 Signal Pathway . Mol Cells . 2018 ; 41 ( 6 ): 575 – 581 . Crossref PubMed Google Scholar
67. Barber MJ , Mangravite LM , Hyde CL , et al. Genome-wide association of lipid-lowering response to statins in combined study populations . PLoS One . 2010 ; 5 ( 3 ): e9763 . Crossref PubMed Google Scholar
68. Kadam UT , Blagojevic M , Belcher J . Statin use and clinical osteoarthritis in the general population: a longitudinal study . J Gen Intern Med . 2013 ; 28 ( 7 ): 943 – 949 . Crossref PubMed Google Scholar
69. Clockaerts S , Van Osch GJVM , Bastiaansen-Jenniskens YM , et al. Statin use is associated with reduced incidence and progression of knee osteoarthritis in the Rotterdam study . Ann Rheum Dis . 2012 ; 71 ( 5 ): 642 – 647 . Crossref PubMed Google Scholar
70. Haj-Mirzaian A , Mohajer B , Guermazi A , et al. Statin use and knee osteoarthritis outcome measures according to the presence of Heberden nodes: results from the Osteoarthritis Initiative . Radiology . 2019 ; 293 ( 2 ): 396 – 404 . Crossref PubMed Google Scholar
71. Lin SM , Wang JH , Liang CC , Huang HK . Statin use Is associated with decreased osteoporosis and fracture risks in stroke patients . J Clin Endocrinol Metab . 2018 ; 103 ( 9 ): 3439 – 3448 . Crossref PubMed Google Scholar
72. An T , Hao J , Sun S , et al. Efficacy of statins for osteoporosis: a systematic review and meta-analysis . Osteoporos Int . 2017 ; 28 ( 1 ): 47 – 57 . Crossref PubMed Google Scholar
73. Williams LJ , Pasco JA , Mohebbi M , et al. Statin and aspirin use and the risk of mood disorders among men . Int J Neuropsychopharmacol . 2016 ; 19 ( 6 ): pyw008 . Crossref PubMed Google Scholar
74. Köhler-Forsberg O , Gasse C , Berk M , Østergaard SD . Do statins have antidepressant effects? CNS Drugs . 2017 ; 31 ( 5 ): 335 – 343 . Crossref PubMed Google Scholar
75. Köhler O , Gasse C , Petersen L , et al. The Effect of Concomitant Treatment With SSRIs and Statins: A Population-Based Study . Am J Psychiatry . 2016 ; 173 ( 8 ): 807 – 815 . Crossref PubMed Google Scholar
76. Salagre E , Fernandes BS , Dodd S , Brownstein DJ , Berk M . Statins for the treatment of depression: A meta-analysis of randomized, double-blind, placebo-controlled trials . J Affect Disord . 2016 ; 200 : 235 – 242 . Crossref PubMed Google Scholar
77. Kim S-W , Kang H-J , Jhon M , et al. Statins and inflammation: new therapeutic opportunities in psychiatry . Front Psychiatry . 2019 ; 10 : 103 . Crossref PubMed Google Scholar
78. Osani MC , Bannuru RR . Efficacy and safety of duloxetine in osteoarthritis: a systematic review and meta-analysis . Korean J Intern Med . 2019 ; 34 ( 5 ): 966 – 973 . Crossref PubMed Google Scholar
79. Wei H , Xiang L , Wayne AS , et al. Immunotoxin resistance via reversible methylation of the DPH4 promoter is a unique survival strategy . Proc Natl Acad Sci U S A . 2012 ; 109 ( 18 ): 6898 – 6903 . Crossref PubMed Google Scholar
80. Halliwell B . Oxidative stress and neurodegeneration: where are we now? J Neurochem . 2006 ; 97 ( 6 ): 1634 – 1658 . Crossref PubMed Google Scholar
81. Szebeni A , Szebeni K , DiPeri T , et al. Shortened telomere length in white matter oligodendrocytes in major depression: potential role of oxidative stress . Int J Neuropsychopharmacol . 2014 ; 17 ( 10 ): 1579 – 1589 . Crossref PubMed Google Scholar
82. Ogłodek EA , Just MJ , Szromek AR , Araszkiewicz A . Assessing the serum concentration levels of NT-4/5, GPX-1, TNF-α, and l-arginine as biomediators of depression severity in first depressive episode patients with and without posttraumatic stress disorder . Pharmacol Rep . 2017 ; 69 ( 5 ): 1049 – 1058 . Crossref PubMed Google Scholar
83. Wigner P , Synowiec E , Czarny P , et al. Effects of venlafaxine on the expression level and methylation status of genes involved in oxidative stress in rats exposed to a chronic mild stress . J Cell Mol Med . 2020 ; 24 ( 10 ): 5675 – 5694 . Crossref PubMed Google Scholar
84. Koretsi V , Kirschneck C , Proff P , Römer P . Expression of glutathione peroxidase 1 in the spheno-occipital synchondrosis and its role in ROS-induced apoptosis . Eur J Orthod . 2015 ; 37 ( 3 ): 308 – 313 . Crossref PubMed Google Scholar
85. Yan J , Guo Y , Fei Y , Zhang R , Han Y , Lu S . GPx1 knockdown suppresses chondrogenic differentiation of ATDC5 cells through induction of reductive stress . Acta Biochim Biophys Sin (Shanghai) . 2017 ; 49 ( 2 ): 110 – 118 . Crossref PubMed Google Scholar
86. Xue J , Min Z , Xia Z , et al. The hsa-miR-181a-5p reduces oxidation resistance by controlling SECISBP2 in osteoarthritis . BMC Musculoskelet Disord . 2018 ; 19 ( 1 ): 355 . Crossref PubMed Google Scholar
87. Jones J , Estirado A , Redondo C , et al. Mesenchymal stem cells improve motor functions and decrease neurodegeneration in ataxic mice . Mol Ther . 2015 ; 23 ( 1 ): 130 – 138 . Crossref PubMed Google Scholar
88. Lean JM , Jagger CJ , Kirstein B , Fuller K , Chambers TJ . Hydrogen peroxide is essential for estrogen-deficiency bone loss and osteoclast formation . Endocrinology . 2005 ; 146 ( 2 ): 728 – 735 . Crossref PubMed Google Scholar
Author contributions
F. Zhang: Methodology, Investigation, Formal analysis, Writing – original draft.
S. Rao: Writing – original draft.
A. Baranova: Writing – original draft.
Funding statement
The authors disclose receipt of the following financial or material support for the research, authorship, and/or publication of this article: the National Natural Science Foundation of China (81471364).
Acknowledgements
We thank members of the Psychiatric Genomics Consortium and other teams, who generously shared the GWAS data.
Supplementary material
Tables showing loci and genes identified by the meta-analysis result, gene-trait associations identified in previous studies, and transcriptome-wide association study results; figure showing quantile-quantile plot of the meta-analysis.
Open access funding
The authors confirm that the open access fee for this study was self-funded.